Asynchronous Temporal Models
Borealis AI is building ATOM (Asynchronous Temporal Model), a foundation model for financial services. Foundation models are AI models trained on large and pervasive datasets and can make predictions that generalize across a variety of tasks and applications. ATOM is trained using large-scale financial datasets, providing it with a breadth of knowledge in financial services.
Recent advancements in Natural Language Processing (NLP) have demonstrated that foundation models pre-trained on large text corpora can achieve superior performance on a wide range of specific NLP tasks. In the same way, the ATOM foundation model’s large-scale pre-training on transactional and other financial data can enable it to deliver superior performance in a variety of financial ML tasks. To draw an analogy to Large Language Models (LLMs) in NLP, we refer to the ATOM Foundation Model as a Large Transaction Model (LTM).
Transaction sequences, such as payment data, stock market trades, loyalty rewards or other client interactions occur at irregularly spaced times. This form of data is known as asynchronous event sequences. The ATOM foundation model specializes in learning from asynchronous event sequences, to maximally utilize the richness of transactional data in financial services.
Borealis AI supports the development and use of cutting-edge science like the ATOM foundation model to inform business and client transactions, all within a responsible AI framework focused on privacy, fairness and transparency.
ATOM in Personal Banking
NOMI, RBC’s award winning product for personal banking, includes a suite of AI tools that help clients better manage their finances. For example, NOMI has the ability to recommend bill payees using customized predictions on our clients’ individual needs. In addition, NOMI Forecast predicts upcoming debits and credits to/from accounts, assisting clients in understanding future cash flows. Most recently, NOMI’s bill-reversal engine, warns clients about having manually input bill payment transactions that seem erroneous. This suite of AI-powered services, rooted in ATOM Foundation Models, work synergistically and in the background of everyday banking interactions.
At its core, NOMI’s predictive capabilities follow the ATOM paradigm, analyzing transaction sequences to build a representation of a client’s transacting behaviour. Whether these are common bill payees, patterns of payroll deposits, subscription service payments, or the typical amounts of Interac e-transfers, ATOM can learn representations that can be used to make helpful predictions to aid our clients.
Machine Learning for a better financial future
The team’s commitment to creating real-world impact through scientific pursuit led to Borealis AI establishing a set of challenging North Star research problems, such as ATOM and building the ATOM Foundation Model. The research team’s work is integral to the projects Borealis AI undertakes in the personal banking space and sits at the core of RBC’s overall innovation strategy.
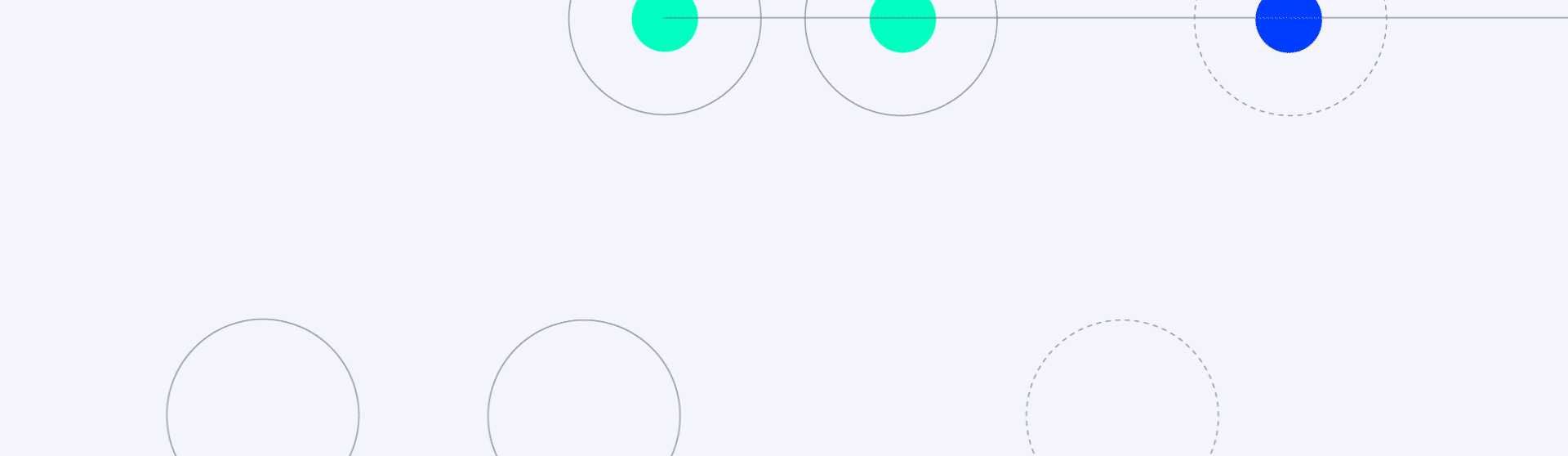
Select ATOM Publications
-
Constant Memory Attention Block
Constant Memory Attention Block
L. Feng, F. Tung, H. Hajimirsadeghi, Y. Bengio, and M. O. Ahmed. Workshop at International Conference on Machine Learning (ICML), 2023
-
Meta Temporal Point Processes
Meta Temporal Point Processes
W. Bae, M. O. Ahmed, F. Tung, and G. Oliveira. International Conference on Learning Representations (ICLR), 2023
-
Ranking Regularization for Critical Rare Classes: Minimizing False Positives at a High True Positive Rate
Ranking Regularization for Critical Rare Classes: Minimizing False Positives at a High True Positive Rate
*M. Kiarash, H. Zhao, M. Zhai, and F. Tung. The IEEE / CVF Computer Vision and Pattern Recognition Conference (CVPR), 2023
-
RankSim: Ranking Similarity Regularization for Deep Imbalanced Regression
RankSim: Ranking Similarity Regularization for Deep Imbalanced Regression
Y. Gong, G. Mori, and F. Tung. International Conference on Machine Learning (ICML), 2022
-
Self-Supervised Time Series Representation Learning with Temporal-Instance Similarity Distillation
Self-Supervised Time Series Representation Learning with Temporal-Instance Similarity Distillation
A. Hajimoradlou, L. Pishdad, F. Tung, and M. Karpusha. Workshop at International Conference on Machine Learning (ICML), 2022
-
Gumbel-Softmax Selective Networks
Gumbel-Softmax Selective Networks
M. Salem, M. O. Ahmed, F. Tung, and G. Oliveira. Workshop at Conference on Neural Information Processing Systems (NeurIPS), 2022
-
Training a Vision Transformer from scratch in less than 24 hours with 1 GPU
Training a Vision Transformer from scratch in less than 24 hours with 1 GPU
S. Irandoust, T. Durand, Y. Rakhmangulova, W. Zi, and H. Hajimirsadeghi. Workshop at Conference on Neural Information Processing Systems (NeurIPS), 2022
Blog
Introducing ATOM: Borealis AI’s research focus on Asynchronous Temporal Models
Report
Real Talk: How Generative AI Could Close Canada’s Productivity Gap and Reshape the Workplace—Lessons From the Innovation Economy
News