Borealis AI conducts research in artificial intelligence for financial services. We are part of RBC, one of the largest banks in the world. At Borealis, we aspire to publish impactful research and build industry-leading products that serve our clients’ needs from the algorithms we create.
North Star core
scientific problems
As part of RBC, we have access to data, problems, and domain experts to drive our research agenda. We organize our research into North Star areas – guiding directions for our work and formulated as a challenging problem we are trying to solve.
Asynchronous Temporal Models (Atom)

The goal of this North Star research direction is to develop novel machine learning technologies for asynchronous time series data, commonly found in banking and other relevant customer-centric domains.
This line of research focuses on training machine learning models in the challenging data environment of partially-observed, multi-source, imbalanced, and asynchronous time series. This research can help build technologies that can leverage the data from multiple channels, but also work for clients that use only a subset of these channels, in a way that respects privacy and upholds principles of responsible AI.
-
Variational Selective Autoencoder: Learning from Partially-Observed Heterogeneous Data
Variational Selective Autoencoder: Learning from Partially-Observed Heterogeneous Data
Y. Gong, H. Hajimirsadeghi, J. He, T. Durand, and G. Mori. International Conference on Artificial Intelligence and Statistics (AISTATS), 2021
-
Point Process Flows
Point Process Flows
*N. Mehrasa, *R. Deng, M. O. Ahmed, B. Chang, J. He, T. Durand, M. Brubaker, and G. Mori. The Conference and Workshop on Neural Information Processing Systems Workshop on Learning with Temporal Point Processes (NeurIPS), 2019
-
Arbitrarily-conditioned Data Imputation
Arbitrarily-conditioned Data Imputation
M. Carvalho, T. Durand, J. He, N. Mehrasa, and G. Mori. Advances in Approximate Bayesian Inference (AABI), 2019
-
LayoutVAE: Stochastic Scene Layout Generation from a Label Set
LayoutVAE: Stochastic Scene Layout Generation from a Label Set
A. Jyothi, T. Durand, J. He, L. Sigal, and G. Mori. International Conference on Computer Vision (ICCV), 2019
-
Learning a Deep ConvNet for Multi-label Classification with Partial Labels
Learning a Deep ConvNet for Multi-label Classification with Partial Labels
T. Durand, N. Mehrasa, and G. Mori. The IEEE / CVF Computer Vision and Pattern Recognition Conference (CVPR), 2019
-
A Variational Auto-Encoder Model for Stochastic Point Process
A Variational Auto-Encoder Model for Stochastic Point Process
N. Mehrasa, A. Jyothi, T. Durand, J. He, L. Sigal, and G. Mori. The IEEE / CVF Computer Vision and Pattern Recognition Conference (CVPR), 2019
Machine Intelligence Beyond Predictive ML (Causmos)
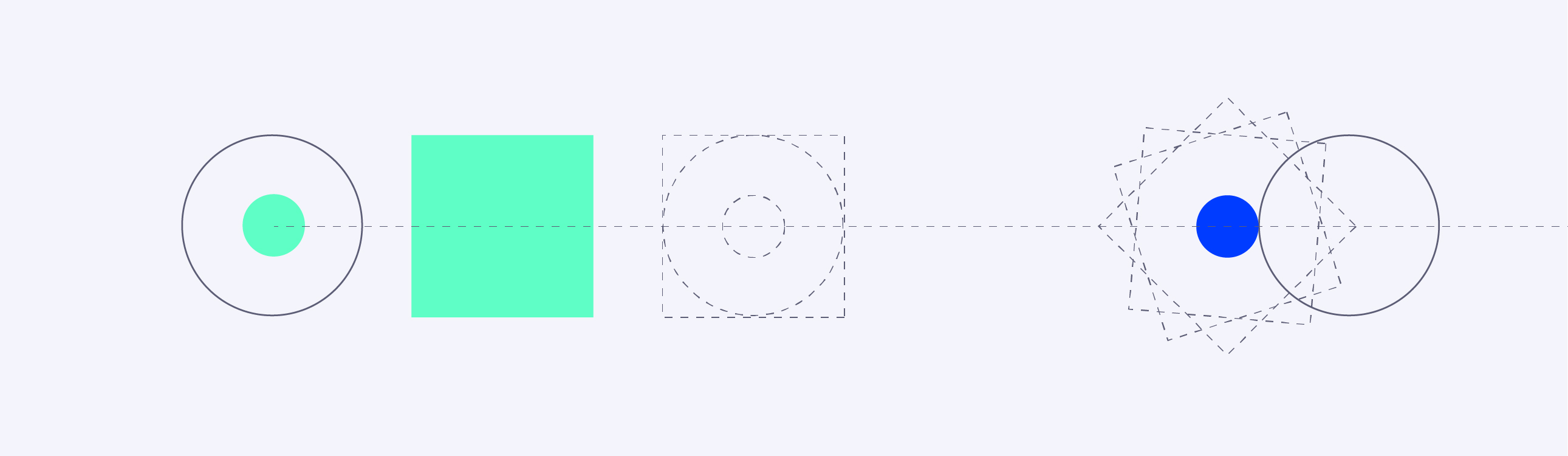
Our AI needs to drive responsible actions and decision-making in rapidly changing environments. With the Causmos program, we aim to build machine intelligence beyond predictive ML for financial services by conducting research in areas such as causality, out-of-distribution (OOD) generalization, reasoning and planning in large language models (LLMs) and reinforcement learning.
In financial services, ensuring our AI systems drive the right decisions for our clients amidst complex, evolving landscapes is crucial. Even when human oversight is integrated as a safety net in mission-critical tasks, AI influences real-world outcomes through human mediation.
The Causmos research program at Borealis AI is dedicated to advancing machine intelligence beyond predictive ML in this domain.
Our focus encompasses different facets and approaches to model actions and their consequences, including reinforcement learning, planning, causal inference, and experimental design. Additionally, we delve into crucial related areas to ensure the safety of actions, such as reasoning, interpretability, adversarial robustness, and out-of-distribution (OOD) generalization.
-
Maximum Entropy Monte-Carlo Planning
Maximum Entropy Monte-Carlo Planning
C. Xiao, R. Huang, J. Mei, D. Schuurmans, and M. Müller. Conference on Neural Information Processing Systems (NeurIPS), 2019
-
TURING: an Accurate and Interpretable Multi-Hypothesis Cross-Domain Natural Language Database Interface
TURING: an Accurate and Interpretable Multi-Hypothesis Cross-Domain Natural Language Database Interface
*P. Xu, *W. Zi, H. Shahidi, A. Kádár, K. Tang, W. Yang, J. Ateeq, H. Barot, M. Alon, and Y. Cao. Association for Computational Linguistics (ACL) & International Joint Conference on Natural Language Processing (IJCNLP), 2021
-
On Variational Learning of Controllable Representations for Text without Supervision
On Variational Learning of Controllable Representations for Text without Supervision
P. Xu, J. Chi Kit Cheung, and Y. Cao. International Conference on Machine Learning (ICML), 2020
-
Optimizing Deeper Transformers on Small Datasets
Optimizing Deeper Transformers on Small Datasets
P. Xu, D. Kumar, W. Yang, W. Zi, K. Tang, C. Huang, J. Chi Kit Cheung, S. Prince, and Y. Cao. Association for Computational Linguistics (ACL) & International Joint Conference on Natural Language Processing (IJCNLP), 2021
-
Object Grounding via Iterative Context Reasoning
Object Grounding via Iterative Context Reasoning
L. Chen, M. Zhai, J. He, and G. Mori. International Conference on Computer Vision Workshop on Multi-Discipline Approach for Learning Concepts at IEEE International Conference on Computer Vision (ICCV), 2019
-
A Cross-Domain Transferable Neural Coherence Model
A Cross-Domain Transferable Neural Coherence Model
P. Xu, H. Saghir, J. Kang, L. Long, A. J. Bose, and Y. Cao. Association for Computational Linguistics (ACL), 2019
-
Adversarial Contrastive Estimation
Adversarial Contrastive Estimation
*A. J. Bose, *H. Ling, and *Y. Cao. Association for Computational Linguistics (ACL), 2018
-
Max-Margin Adversarial Training: Direct Input Space Margin Maximization through Adversarial Training
Max-Margin Adversarial Training: Direct Input Space Margin Maximization through Adversarial Training
G. W. Ding, Y. Sharma, K. Lui, and R. Huang. International Conference on Learning Representations (ICLR), 2020
Non-cooperative learning in competing markets (Photon)
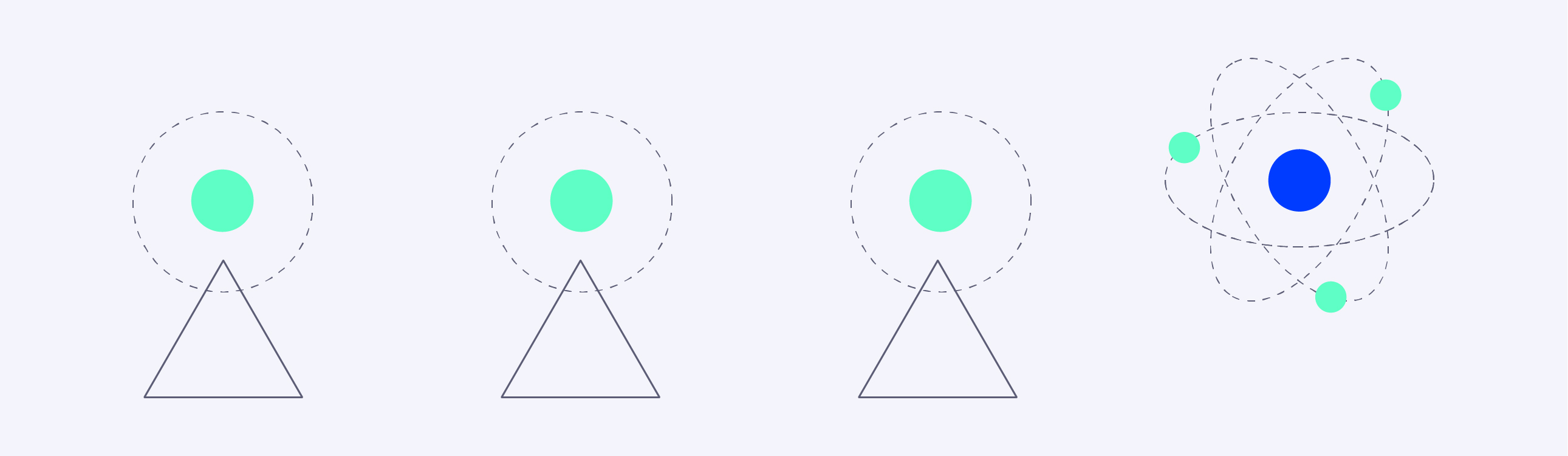
We build models for Capital Markets data, where particular challenges include low signal-to-noise ratio, structured prediction, and game theoretic impacts from decisions we make.
Globally, Capital Markets have gone through a paradigm shift towards complete automation through Artificial Intelligence, turning it into a highly competitive area at the intersection of statistical models from various branches of machine learning.
We believe that a principled understanding of the interactions between statistical models that operate in a common environment will soon be a key success factor for leaders in the field. The Photon north star research team plans to approach this challenge from two angles:
Atomistic: a symptom-based research stream focusing on novel solutions to challenges that are a direct consequence of a competing market: large data, high noise, non-stationary dynamics, and constrained environments
Holistic: a system-based research stream focusing on a meta-level framework for holistic properties of a competing market: local stability, asymptotic behaviour, perturbation theory, and adversarial robustness.
-
Continuous Latent Process Flows
Continuous Latent Process Flows
R. Deng, M. Brubaker, G. Mori, and A. Lehrmann. Conference on Neural Information Processing Systems (NeurIPS), 2021
-
Efficient CDF Approximations for Normalizing Flows
Efficient CDF Approximations for Normalizing Flows
C.S. Sastry, A. Lehrmann, M. Brubaker, and A. Radovic. Transactions on Machine Learning Research (TMLR), 2022
-
Generating Videos of Zero-Shot Compositions of Actions and Objects
Generating Videos of Zero-Shot Compositions of Actions and Objects
M. Nawhal, M. Zhai, A. Lehrmann, L. Sigal, and G. Mori. The European Conference on Computer Vision (ECCV), 2019
-
Agent Forecasting at Flexible Horizons using ODE Flows
Agent Forecasting at Flexible Horizons using ODE Flows
A. Radovic, J. He, J. Ramanan, M. Brubaker, and A. Lehrmann. International Conference on Machine Learning Workshop on Invertible Neural Nets and Normalizing Flows (ICML), 2021