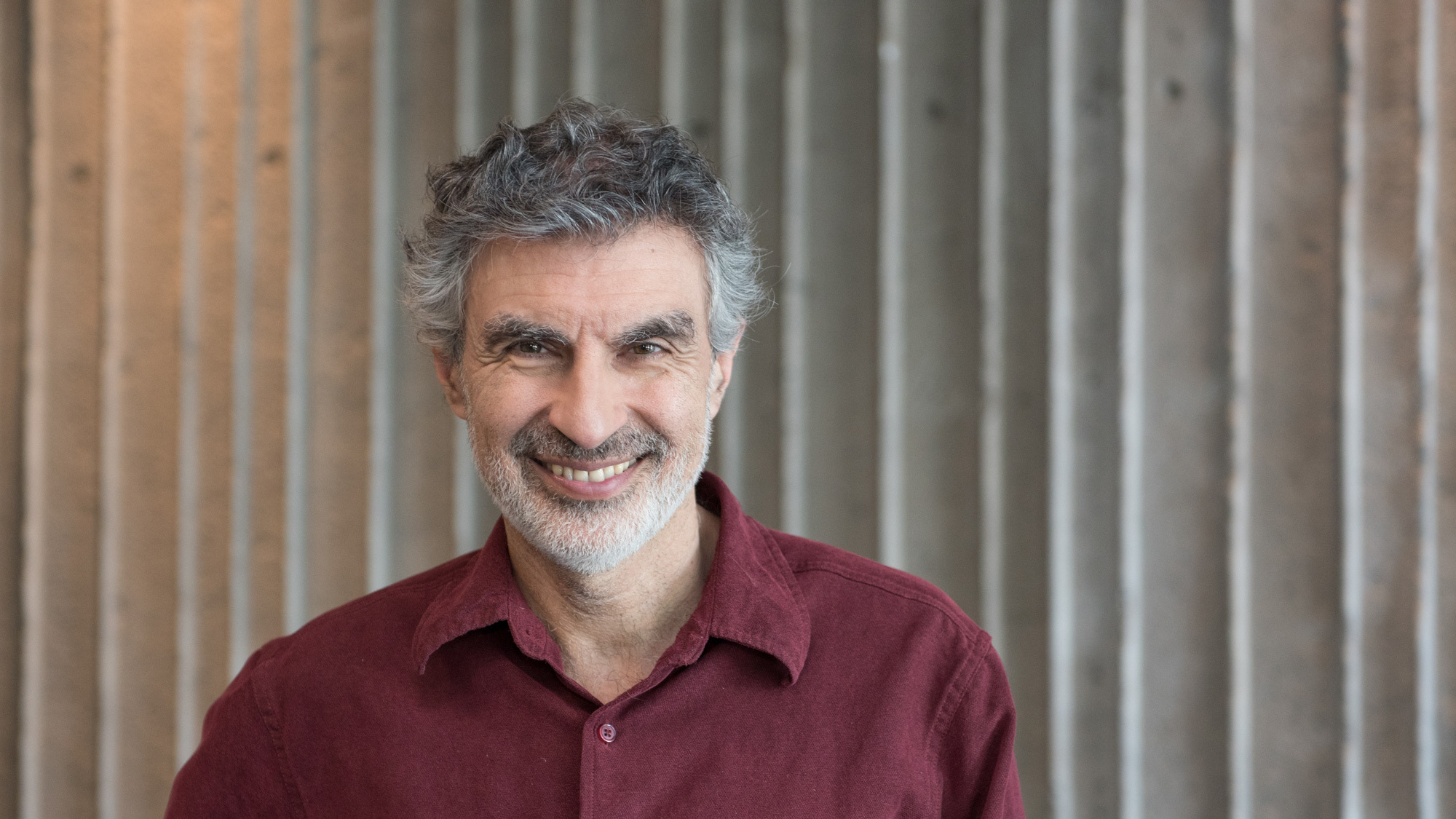
As the pace of evolution in AI and Machine Learning accelerates, we talk with one of the ‘Godfathers of AI’, Professor Yoshua Bengio, Full Professor at Université de Montréal and the Founder and Scientific Director of Mila – Quebec AI Institute, about the link between human brains and AI, key future research areas and risks to avoid.
The views expressed in this article are those of the interviewee and do not necessarily reflect the position of RBC or Borealis AI.
Do machines and humans learn in the same way?
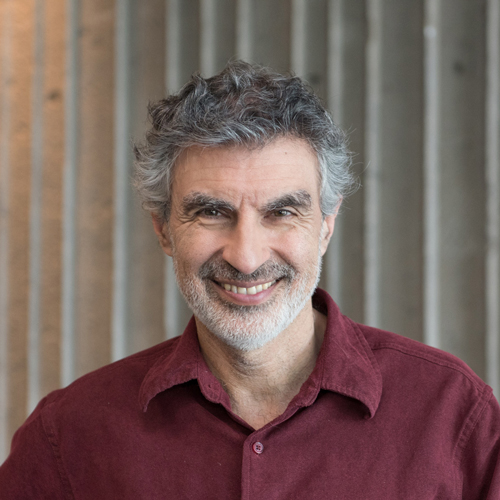
Humans are good at something because we practice it through thinking, abstraction and incorporating anything we study or experience. Machines are the same. They need to practice a lot.
Consider the notion of inductive bias. Basically, evolution has sculpted the way we learn as humans. Evolution has selected all of these minute changes to the way we learn over hundreds of millions of years. But when we build AI systems, we don’t have that luxury. So we tend to do it by using our insights about computing, intelligence, logic, about programming – anything we can put our hands on that might help us design these learning machines.
The bottom line is that both humans and machines learn by making small changes, one at a time. That’s the practice part. In the same way, we make small changes to, say, the parameters of a neural net to find improvements. And deep learning tends to lean towards a mathematical concept we call the gradient – essentially, what is the smallest change you can make to obtain the largest improvement in how the machine behaves on a particular task.
So is it worth studying the brain when developing AI?
There are certainly many connections between human brains and machine learning. Both analyze inputs using computations performed by neurons. In the brain, these are biological neurons that take in some signals coming from other neurons and send their signal to a bunch of other neurons. In deep learning, it’s very similar – except they are not real neurons but rather some coarse analogy inspired by the brain.
But they are certainly correlated. Say we train neural nets with the objective to do computer vision, and we look at the patterns of activity of these artificial neurons in our machine. Then we ask a monkey to do the same visual task and measure the activity of the neurons in the monkey’s brain; we find they are very correlated and predictive of each other.
In the past, machine learning got much inspiration from biology, but that relationship is now driving research in neuroscience in the other direction, with neuroscientists looking at what deep learning researchers are inventing and thinking of it as potential inspiration for theories about what is going on in the brain.
How could work on causality influence the future of AI?
Causality is very useful for humans. It allows us to plan our actions properly. If you don’t take into account the causal structure of the world, you might come to the conclusion that wet ground causes rain. If you just look at the statistical dependency between those two variables – rain and wet ground – it doesn’t tell you which caused which. A causal model is really useful if you want to do things in the real world to achieve the intended effects.
The other reason we are interested in causality is because it helps with generalization to new settings. I’ve been driving in North America all my life. But when I go to the UK, I rent a car, and that means I need to drive on the left, not on the right. It’s one little detail, but it has massive consequences. Human brains can somehow foresee those consequences and can retrain in a very short amount of time. We’d like to have that kind of capability in machines so that when the world changes – and it changes all the time – they can generalize much better to new situations.
Are generative AI and large language models science or sorcery?
Right now, it’s both. I think the issue is that current deep learning – including the large language models – is implementing what physiologists call System One thinking. That’s the intuitive thinking we do when we act without thinking. When you add in human reason, we are talking about System Two thinking.
So let’s go back to my example of driving in the UK for the first time. If you just use your intuitive system without any reasoning, you are going to make some pretty bad mistakes. But that’s not what happens for us. Instead, you are going to be driving very carefully, paying attention and thinking about what you are doing and what the consequences are of your actions. And you are going to retrain your habitual system – your System One thinking – to be consistent with your reasoning so that, eventually, you can drive in London without having to think about the changed traffic rule all the time.
There’s research around the world right now, including in my group and in Mila and at other institutes, to think about how humans do things differently than current deep learning systems and how we can make them reason more like humans with System Two thinking.
How can we ensure that scientific and technological advances around AI benefit humans?
That’s a great question, and I wish I knew the answer. Here’s the way I see it at an abstract level – humans build tools. We are really good at it. We started with rocks. We used them to build houses. And we used them to kill each other. We started building more and more powerful tools. And, for a long time, the worst use case wasn’t so bad since our tools were pretty limited in the damage they could do. Then we got to the point of building tools that could destroy everyone, like nuclear weapons.
I think AI is along those lines. The more powerful it gets, the more it could really help us with the challenges we face, whether that’s climate change, pandemics, cancer, you name it. But AI could also be used in nefarious ways that could be really, really bad. Like destroying democracy, for example. Or AI-enabled weapons like killer drones. It could be scary, and there is probably worse stuff that’s going to show up as we make those machines smarter and people explore their uses for their own benefit.
So what can we do about it? I think we need to change our society. The least we can do is have better regulations that set the limits to what we consider to be morally and socially acceptable and what is not. But I think that will require international coordination to a level that is much, much greater than what we currently have. I think we need to move slowly and thoughtfully.
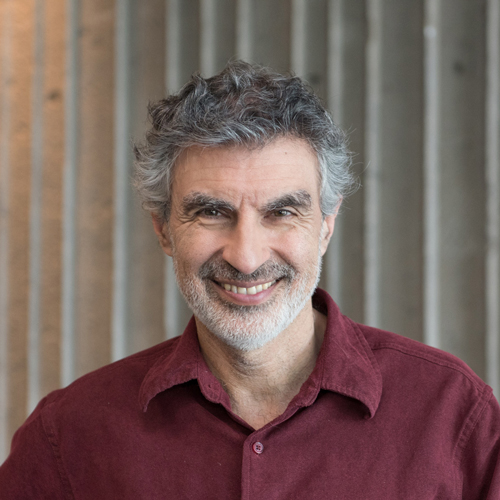
About Prof. Yoshua Bengio
Yoshua Bengio is a full Professor in the Department of Computer Science and Operations Research at Université de Montreal, as well as the Founder and Scientific Director of Mila and the Scientific Director of IVADO. Considered one of the world’s leaders in artificial intelligence and deep learning, he is the recipient of the 2018 A.M. Turing Award with Geoff Hinton and Yann LeCun, known as the Nobel Prize of computing. He is a Fellow of both the Royal Society of London and Canada, an Officer of the Order of Canada, a Knight of the Legion of Honor of France, and a Canada CIFAR AI Chair.
Article photo credit: Amelie Philibert 2022
Advancing Responsible AI
Responsible AI is key to the future of AI. We have launched RESPECT AI hub to share knowledge, algorithms, and tooling to help advance responsible AI.
Visited RESPECT AI hubNews