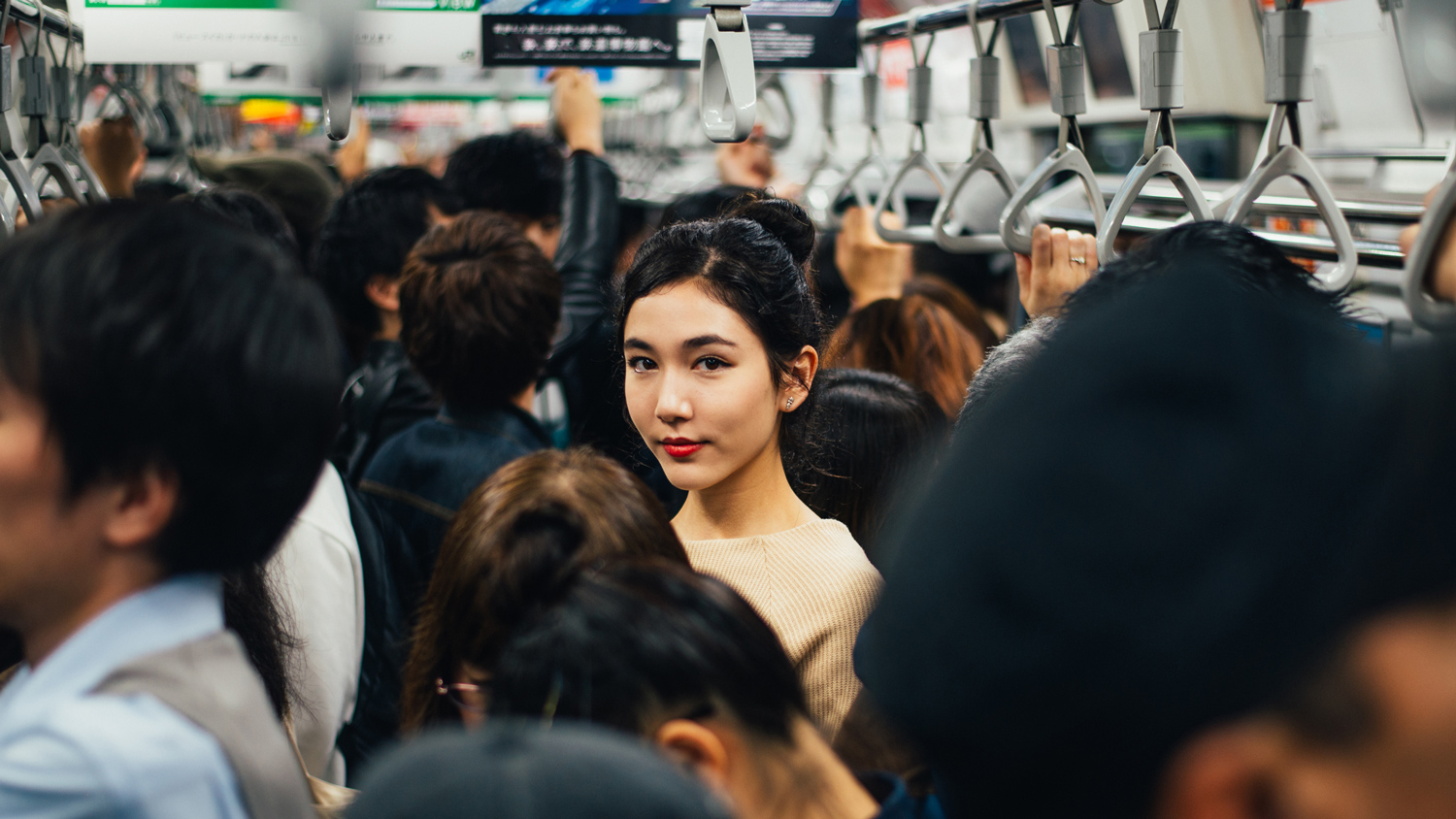
The regulatory environment surrounding AI and Machine Learning is rapidly evolving as new AI systems and tools – like ChatGPT – shine the spotlight on potential risks. In this article, we talk with Carole Piovesan, Managing Partner at INQ Law, about where the regulation is going and what impact it might have on AI developers and their organizations.
The views expressed in this article are those of the interviewee and do not necessarily reflect the position of RBC or Borealis AI.
Can you paint us a picture of the current regulatory landscape regarding AI globally?
There are different approaches to the regulation of AI being taken around the world. In the US, for example, the US National Institute for Standards and Technology released its AI Risk Management Framework, which provides a voluntary, self-regulatory framework by establishing guidance on how to develop and implement a risk-based approach to AI development and deployment. The EU, on the other hand, has a draft law – the EU Artificial Intelligence Act – which is much more prescriptive by establishing specific requirements for the development and deployment of ‘high risk’ AI throughout the AI lifecycle. What qualifies as ‘high risk’ is described in the appendix of the proposed law. And there are specific measures that organizations need to put in place to properly assess the data, to validate and verify models, and to monitor the use of the high-risk AI system in an iterative, ongoing manner.
Canada is somewhere in the middle. Bill C27 – The Artificial Intelligence and Data Act (AIDA) – provides higher-level requirements for the development and deployment of AI systems that are considered ‘high impact’ (at the time of writing, the definition of ‘high impact’ was described in the AIDA Companion document). AIDA is still draft. It is not as prescriptive as the EU’s draft law and much of the law is left to be defined in subsequent regulation.
Has the release of consumer-grade Large Language Models sharpened regulator focus?
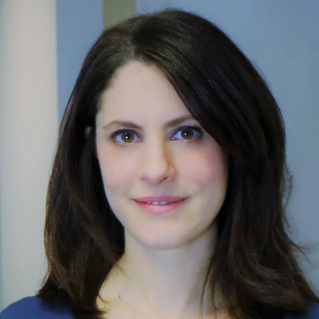
We’ve been talking about AI ethics for years, and AI ethics has fed into what the current regulations and high-level principles look like. Models like ChatGPT are now also catalyzing the conversation at scale. I think ChatGPT has really allowed people to understand the potential and power of AI, both the positive and the potential harms.
When thinking about operationalizing a tool like ChatGPT in business practices, one of the biggest considerations is to understand its limitations. OpenAI has been very clear that this is not a perfect technology. It is based on information that is limited in time. It’s not comprehensive in its responses. And it’s not always accurate either. We need to remember that it’s a learning model, so the outputs are highly correlated to the inputs. Feed it a lot of harmful information and you can expect a harmful output.
I often talk about the Myth of AI Neutrality and the Myth of AI Perfection. These systems are not neutral, and they are not perfect. They have an accuracy and a reliability rate you need to understand when you are using them. ChatGPT is really bringing AI to life, but it is also forcing us to touch and experience some of the harmful implications.
So is regulation really what is needed?
Regulation helps us understand where there are potential harms and what actions need to be taken to be accountable for – and to mitigate – those potential harms. Government has a responsibility to regulate the harms, require a responsible approach from organizations, and to provide guidance related to how you do it. Internationally, there is still some debate about who is best placed to regulate AI, however. Some argue that you need a standalone AI supervisory authority similar to what you see around data protection. Spain has come out with its national strategy on AI and is already looking to stand up an AI supervisory authority.
In Canada, the AIDA proposes that an AI and Data Commissioner post be developed within Innovation, Scientific and Economic Development Canada. That has sparked questions about whether the Ministry has the right resources and requisite team skills and knowledge to effectively regulate this type of law.
Will these current and proposed regulations achieve their goal of reducing harm?
Regulation will help define accountability mechanisms for AI. Going forward, every organization building and/or deploying high-impact AI systems will need to implement an effective AI governance program to augment existing data governance efforts.
An AI governance program should start with education and culture to prevent a ‘checkbox’ approach to compliance, which doesn’t necessarily mean that you are mitigating potential harms effectively. You may end up beefing up your compliance team, just to check a bunch of boxes without actually achieving your objective.
Regulation is designed to encourage organizations to change their culture, to improve education, to enhance digital literacy across the organization, and to take a more responsible approach to what they are building. It’s about mitigating the actual downstream harm.
Some of the regulations may still be uncertain. But if you take a step back, you see the same themes coming up again and again – it needs to be a lifecycle approach. It needs to start with ethics by design. We have to think about the organizational culture and the organizational infrastructure to support responsible innovation and responsible design.
These aren’t new principles – we’ve been dealing with them in the context of personal information and privacy law for some time – but now we are extending it into the use of a specific technology that is attracting a ton of attention and a greater degree of oversight from regulators. It’s all about making sure you have responsibility baked into all aspects of the lifecycle.
About Carole Piovesan, JD, MSc (Hons)
Carole is Managing Partner at INQ Law, focusing her practice on privacy, cyber incident response, data governance and artificial intelligence (AI) risk management. She regularly counsels clients on a wide range of matters related to privacy, cyber readiness and breach response, data governance, ethical AI and responsible innovation. Carole plays an active role in shaping data law, policy and standards in Canada and globally. She is a member of the OECD.AI Policy Observatory and has contributed to numerous organizations, including in the Global Partnership on AI, the Global Task Force to Promote Trusted Sharing of Data at the German Marshall Fund of the United States, the Standards Council of Canada’s Data Governance Collaborative, and the National Advisory Council on AI in Healthcare.
👋 We're Hiring!
Borealis AI offers a stimulating work environment and the platform to do world-class machine learning in a startup culture. We're growing the team, hiring for roles such as a Sr. Product Manager, Machine Learning Researcher and Research Engineer.
View all jobs