
Much has been written about the risks of generative AI. In this article, we talk with Dr. Graham Taylor, Canada Research Chair in Machine Learning at the University of Guelph and Canada CIFAR AI Chair and Research Director at the Vector Institute for Artificial Intelligence, about the potential benefits of generative AI and how it might change the way humans and machines collaborate.
The views expressed in this article are those of the interviewee and do not necessarily reflect the position of RBC or Borealis AI.
Is generative AI hype or revolution?
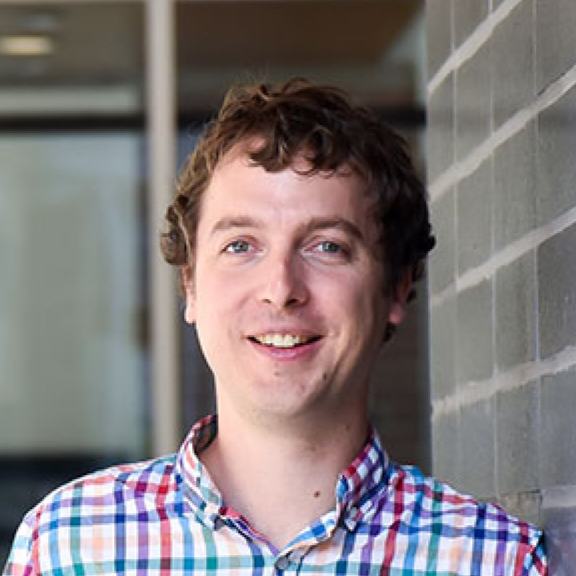
There’s no doubt these are exciting times in terms of AI capabilities research. And I understand why there is so much hype around all of this. I think the excitement we are seeing today is really driven by two things: usability and accessibility. In releasing ChatGPT, OpenAI has really made the user interface very smooth and easy to use. And they have allowed the world to access these models in very different ways.
Fundamentally, though, I’m not sure we can call it a technological revolution rather than a sociological revolution. The scaling of these models is truly impressive. But it reminds me of when we took 1980s and 1990s technologies like convolutional neural nets and recurrent neural nets and scaled them up after the release of ImageNet about 10 years ago.
We hear a lot about the risks of generative AI. What are some of the potential benefits?
I’m personally very excited about design problems where researchers currently focus on a narrow search space and then iterate over a few potential designs. I think generative AI is going to vastly widen the search spaces we can consider. And I think that will quickly lead to more physical realizations of generative design in areas like medicines and materials discovery.
There are serious social and environmental problems facing the world today. We need better medicines. We need better vaccines. We need more efficient materials to help us transition into a greener economy. I believe we have an opportunity to align AI with human values by prioritizing the development of AI systems that serve these societal needs.
What role will humans play in all of this?
AI is a powerful collaborator. But it is not a substitute for human intelligence and creativity. That type of collaboration between humans and machines works best when it is bidirectional – so the AI and the human are working together in an augmented approach. It’s not replacing the human.
I think that is one of the reasons that tools like ChatGPT have been so successful. They were designed to be collaborative. The interaction is all very natural through a chat-based system. OpenAI achieved this by using supervised fine-tuning from demonstration data (SFT) and reinforcement learning from human feedback (RLHF), training it specifically to be helpful and human-like in its interactions. I think that’s an important lesson to remember as we create more collaborative human-AI systems.
What does that mean for the way humans are trained going forward?
That’s a very interesting open debate right now. Take prompt engineering, for example. ChatGPT may be very intuitive to use, but the large language models that came before it and many of the open LLMs coming onto the scene now require you to think very carefully about how you structure the prompts you give them. So there is a growing discipline around prompt engineering. Does that mean everyone in the industry should be going out and taking a course on prompt engineering? A recent set of interactive labs offered by the Vector Institute on prompt engineering was extremely popular among our sponsors.
But, again, ChatGPT and its associated strategies like SFT and RLHF are changing all of that. As one of my Vector Institute colleagues, Jimmy Ba, recently noted at a Creative Destruction Lab, “The latest programming language is English.” So there is an open debate about how important prompt engineering will be in the future. New disciplines may pop up and then be abated rather quickly as the technology and capabilities evolve.
One area of Responsible AI that is less frequently discussed is environmental. How does AI influence the environment?
Obviously, the deployment of these massive AI systems requires a lot of computational power. The bigger we make the models, the more energy is needed to run them. And while we continue to make good strides in making the hardware more efficient, demand growth does not seem to be slowing down. So an important part of my research program relates to reducing the computational requirements associated with doing deep learning.
On the other side of the coin, I’m also working on biodiversity applications – areas like biodiversity monitoring and trying to detect species at risk from images and DNA. Those could have a very positive outcome for biodiversity. The Canadian Institute for Advanced Research (CIFAR) has also recently convened a working group of Canada CIFAR AI Chairs who, like me, are interested in AI for energy and the environment.
Where can developers and industry leaders go to better understand the intersection between AI and human values?
There are lots of venues focusing on this right now. I’m co-director of the Center for Advancing Responsible and Ethical AI out of the University of Guelph. And initiatives from private enterprises, like Borealis AI’s Responsible AI program, certainly offer lots of perspectives and insights.
Two great books I would recommend are The Alignment Problem by Brian Christian – it’s a very accessible introduction to aligning AI systems with human values. And on the more technical side, Prediction Machines, a book by Ajay Agrawal, Avi Goldfarb and Joshua Gans. They also have a follow-up book called Power and Prediction which is very good. Avi will be speaking about this book at the University of Guelph on July 6.
Ultimately, if you really want to learn about AI and these difficult questions around ethics and responsibility, you probably want to turn to the Pan-Canadian AI Centers. That’s why they were established, and they are doing a great job leading the way here and around the world. The Alberta Machine Intelligence Institute (Amii) has recently released a Principled AI Framework, and the Vector Institute has one in the works as well.
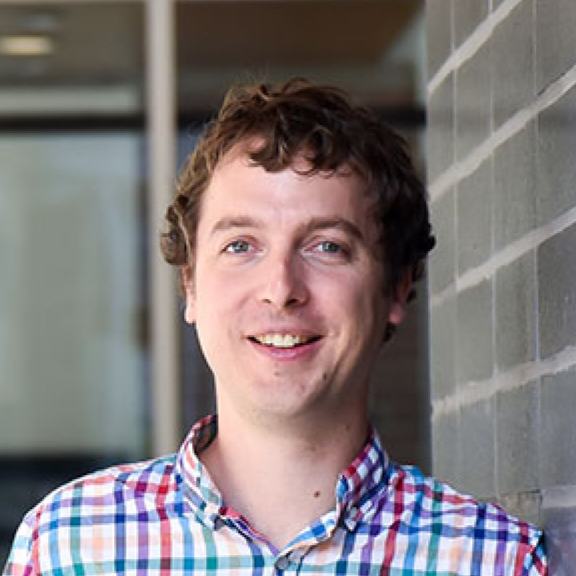
About Graham Taylor
Graham Taylor is a Professor of Engineering at the University of Guelph, a Canada CIFAR AI Chair, an Academic Director of NextAI, a Research Director and a Faculty Member of the Vector Institute for Artificial Intelligence. His research spans a number of topics in deep learning. He is interested in open problems such as how to effectively learn with less labeled data and how to build human-centred AI systems. He also pursues applied projects with global impact: such as using computer vision to mitigate biodiversity loss. He co-organizes the annual CIFAR Deep Learning Summer School and has trained more than 80 students and staff members on AI-related projects.
He will also be giving a talk, “Machine learning for biodiversity,” on June 19 at 3:45 pm PT at the 2nd Workshop on Learning with Limited Labelled Data for Image and Video Understanding, a CVPR 2023 workshop organized in part by Borealis AI’s Machine Learning Researcher, He Zhao.
Advancing Responsible AI
Responsible AI is key to the future of AI. We have launched RESPECT AI hub to share knowledge, algorithms, and tooling to help advance responsible AI.
Visited RESPECT AI hubNews