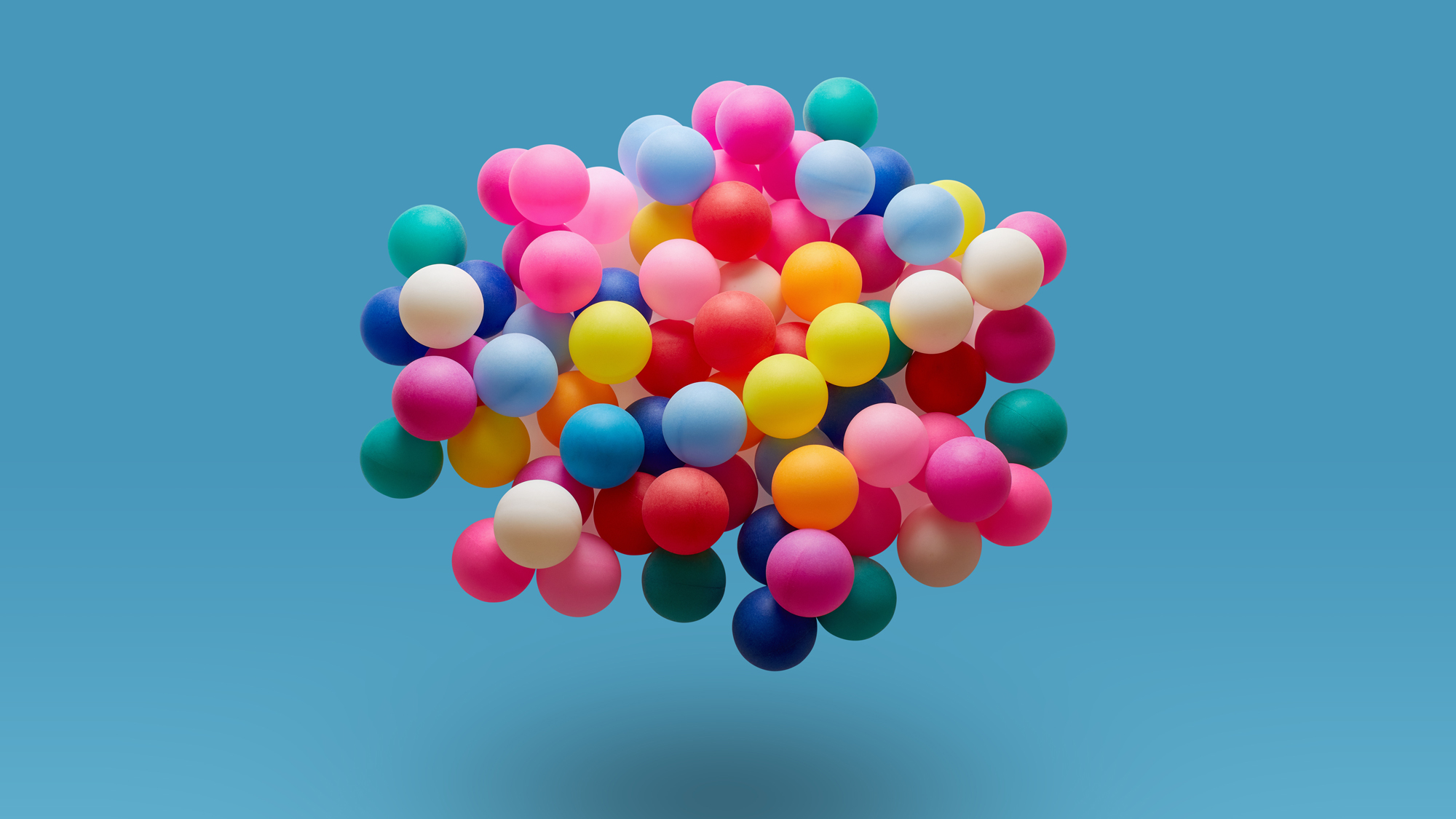

Breaking Down Silos with Responsible AI
How can developers, researchers, and business leads can work together to build a comprehensive Responsible AI system that helps solve real problems and ensure responsible adoption of AI?
The recent panel discussion Borealis AI hosted on LinkedIn, set out to address some of these questions. Titled “Breaking Down Silos in Responsible AI,” the event was led by the host Jaime Trivino, Borealis AI’s senior talent acquisition lead, with Christopher Srinivasa, senior machine learning research team lead, Alex Scott, group business developer, and Dominique Payette, responsible AI strategy lead, joining the event as panelists.
Safe AI and explainability, defined by Payette as “processes and methods that allows human users to comprehend and trust the results and output created by machine learning algorithms” sits at the core of responsible AI adoption. Scott noted that, “If we’re going to be making critical decisions, those models should be explainable. Customers have a right to understand how their data was being used, and how the AI was making decisions that have an impact on them.”
Srinivasa followed up on Scott’s input with a suggestion to consider the implications of using third-party vendor models, where internal characteristics of the models may not be known, but the need still exists to be able to provide some level of explainability. “This is the type of area where there’s high business applicability. We’re looking into alternative methods to help explain the internal workings of those models or being able to look at things like feature importance, to help assess and calibrate AI models,” he said.
A regulated industry such as banking can be a positive influence when it comes to responsible AI adoption.
While ensuring responsible adoption of AI should be paramount for every industry, the sector that could be one of the most prepared and best positioned to take on this challenge is banking. “Banking is already submerged with different policy frameworks and governance model governance is not new at banks. Banks have used models for years,” she said, pointing out that risk management, governance over data, and privacy is already common across financial institutions.
Borealis AI has launched RESPECT AI platform organized around five pillars: Robustness, Fairness, Model Governance, Data Privacy, and Explainability. Through RESPECT AI, Borealis AI is able to contribute research, share open-source code and tooling with the wider community, and bring together experts from a variety of organizations and backgrounds to address some of the common challenges when it comes to responsible AI adoption.
Borealis AI has also published research on novel testing methods of existing ML models, such as fAux: Testing Individual Fairness via Gradient Alignment. fAux method makes it possible to test models for discriminatory predictions with low model overhead and provide actionable feedback developers can use to ensure their models are safe. “This could be useful in a variety of context and it can be as simple as setting parameters around someone, for example, getting a loan and not getting a loan, and then looking at gradient signals in both of these models and comparing them to one another,” Srinivasa said. “This type of work is how we contribute ideas to the research community about alternative ways that we can sort of jump over some of the barriers to responsible AI adoption developers currently face on the technical side.”
Breaking down the silos can go a long way to help ensure a streamlined Responsible AI strategy. As Scott said, “When thinking about a project that might involve machine learning, organizations should be talking about what the responsible thing to do here is, from business requirements through to model development and implementation.” This means taking the time to figure out how the model is going to be used, who it’s going to touch, and how it’s going to impact the users.
Businesses looking to build and implement Responsible AI systems could begin the process by breaking down the silos: consider the context and various stakeholders across multiple disciplines, gathering everyone’s input on what they think the right way forward would be, and how responsible AI should be integrated into everyone’s workflow.
Borealis AI is growing. Please consider joining the team!
News
Always learning: Borealis AI and the DLRL Summer School
News