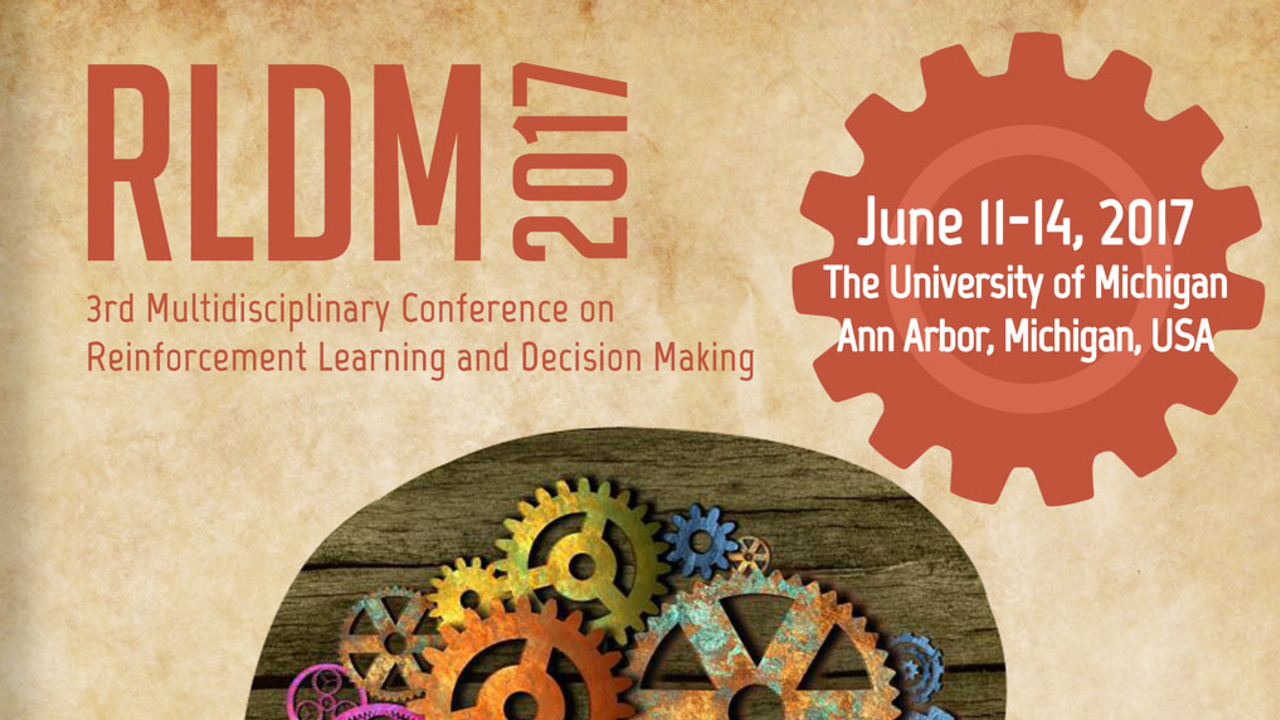
The 2017 Reinforcement Learning and Decision Making (RLDM) conference, informally known as the Gathering of the Brains™, is a place where some of the world’s leading neuroscientists and psychologists join up with the machine learning community to advance what we know about the human brain’s connection to AI neural networks.
This year, Borealis AI shipped a team of delegates down to Ann Arbor, Michigan for a week of fascinating workshops, papers, and bridge building. Here are some of the trends that stood out to us.
Meta-learning in machine learning conforms to observations in neural science
Matthew Botvinick‘s tutorial confirmed the neural network’s increasing link to neuroscience by demonstrating corresponding phenomena between meta-learning algorithms and human brain functionalities. Meta-learning, or “learning how to learn,” is essentially about finding the pattern of how to extract patterns through the algorithms used to train the network. In essence, it’s a higher level of automation.
Why is this interesting? Until now, the early-stage meta-learning algorithms have only been able to tackle small-scale problems. By showing there are similar phenomena to neural networks in the brain, it gives confidence that the algorithms will eventually be able to work on larger-scale problems that have so far eluded us.
Deep learning and reinforcement learning have more commonalities than ever before
The community has been trying to use deep neural networks to replace components in reinforcement learning systems. Some examples of the experiments they’ve successfully run have shown that aspects like objective function, policy, and value function can all be applied to the deep.
Why is this interesting? These continual discoveries demonstrate wide-ranging implications for reinforcement learning and how RL components will only be improved by such deep learning techniques. In tangible terms, this translates into better performance, and the enablement of harder, larger-scale, problems. It’s another leap forward in our progress, opening up new possibilities.
More work around learning to cooperate and communicate
There’s been a lot of momentum recently around training agents to cooperate on their own without giving the agents specific communication instructions. What this means is you build the structures, like, for example, this series of green and red balls from OpenAI, but don’t tell them how to communicate specifically, then you watch as natural patterns emerge during that process.
Why is this interesting? It extends the intelligence behaviour from a single agent to multiple agents in an autonomous way, creating room for more complex patterns.
The overarching trend, however, is how quickly AI is now moving and how fascinating it is to witness these different methods unfold.
Click on the gallery below for our full RLDM album.
Blog
ICML 2020 Roundup
Blog