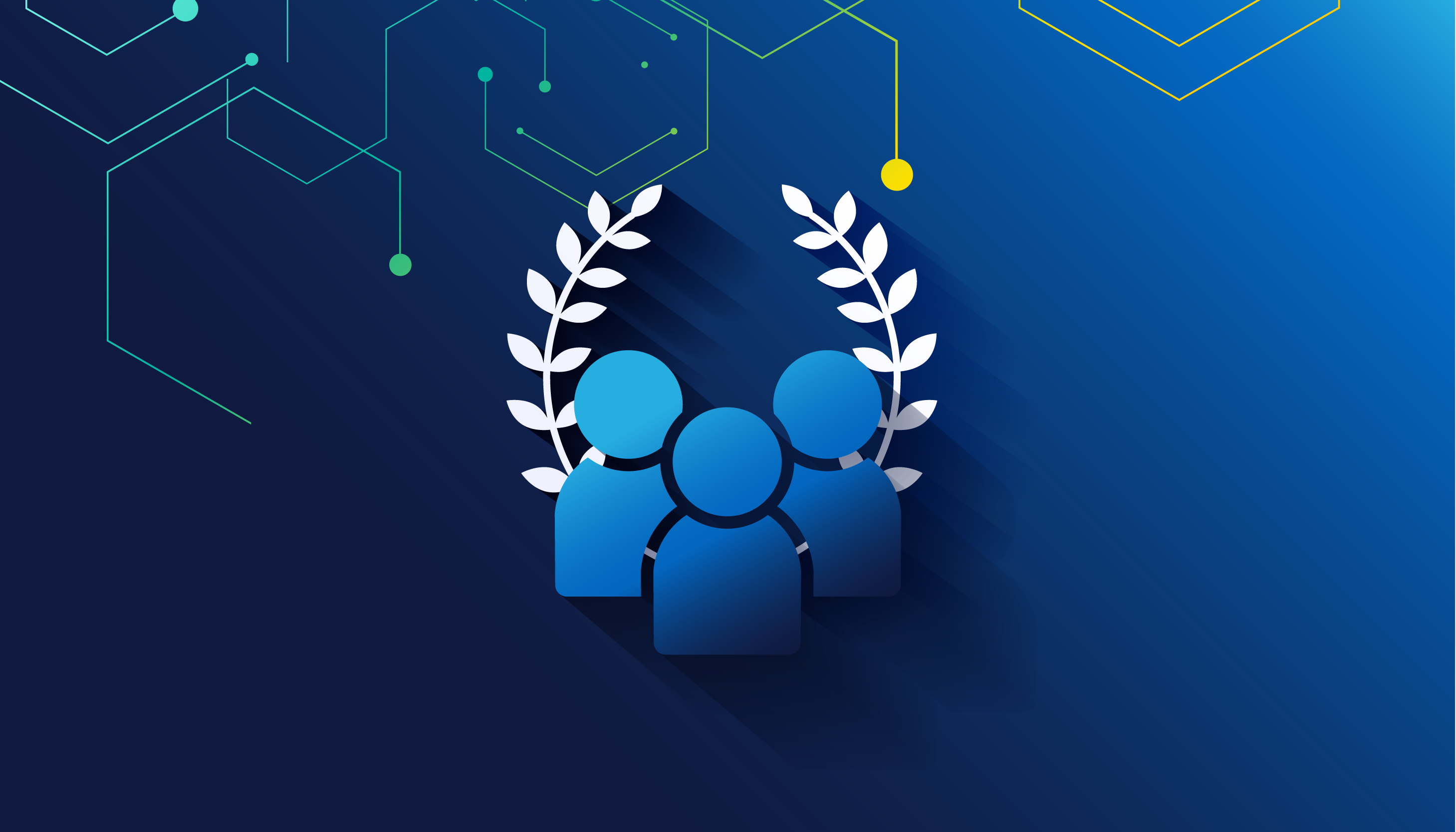
Meet the ten recipients of this year’s fellowships.

Anna Golubeva
School: Perimeter Institute for Theoretical Physics, University of Waterloo.
Research areas: Theoretical physics.
Research topic: Machine Learning for physics and physics for machine learning.

Chenyang Huang
School: AMII, University of Alberta.
Research areas: Machine learning, deep learning and natural language processing.
Research topic: Towards empathetic conversational AI.

Elahe Rahimian Najafabadi
School: Concordia Institute for Information System Engineering (CIISE), Concordia University, Montreal.
Research areas: Deep learning in health domain applications.
Research topic: Designing optimal deep neural networks for hand gesture recognition and force prediction, and developing domain adaptation algorithms for time-domain features.

Guodong Zhang
School: University of Toronto.
Research areas: Machine learning.
Research topic: Interplay between optimization, generalization and uncertainty of deep learning.

Ibtihel Amara
School: Centre for Intelligent Machines (CIM), McGill University.
Research areas: Computer vision and artificial intelligence.
Research topic: Towards building reliable deep neural network.

Michael Skinnider
School: University of British Columbia.
Research areas: Deep learning, chemistry, natural language processing, artificial intelligence, generative models, mass spectrometry.
Research topic: Automated discovery of unknown molecules using deep neural networks.

Reyhane Askari Hemmat
School: Mila, Université de Montréal.
Research areas: Optimization and deep learning.
Research topic: A dynamical systems perspective into game optimization.

Sandeep Subramanian
School: Mila, Université de Montréal.
Research areas: Natural language processing and deep learning.
Research topic: Learning and modeling neural representations of text.

Sedigheh Zolaktaf
School: Artificial Intelligence and Algorithms laboratories at the University of British Columbia.
Research areas: Stochastic processes – neural networks – DNA computing.
Research topic: Mean first passage time and parameter estimation for continous-time Markov Chains.

Xiaohong Liu
School: McMaster University.
Research areas: Deep learning, watermarking, steganography, information-theoretical principles.
Research topic: New deep neural network architectures for blind image watermarking based on the information-theoretic principles.
News
Borealis AI Announces the Fellows of 2020
News
Dr. Jakob Foerster on self-play and zero-shot coordination in Hanabi
News