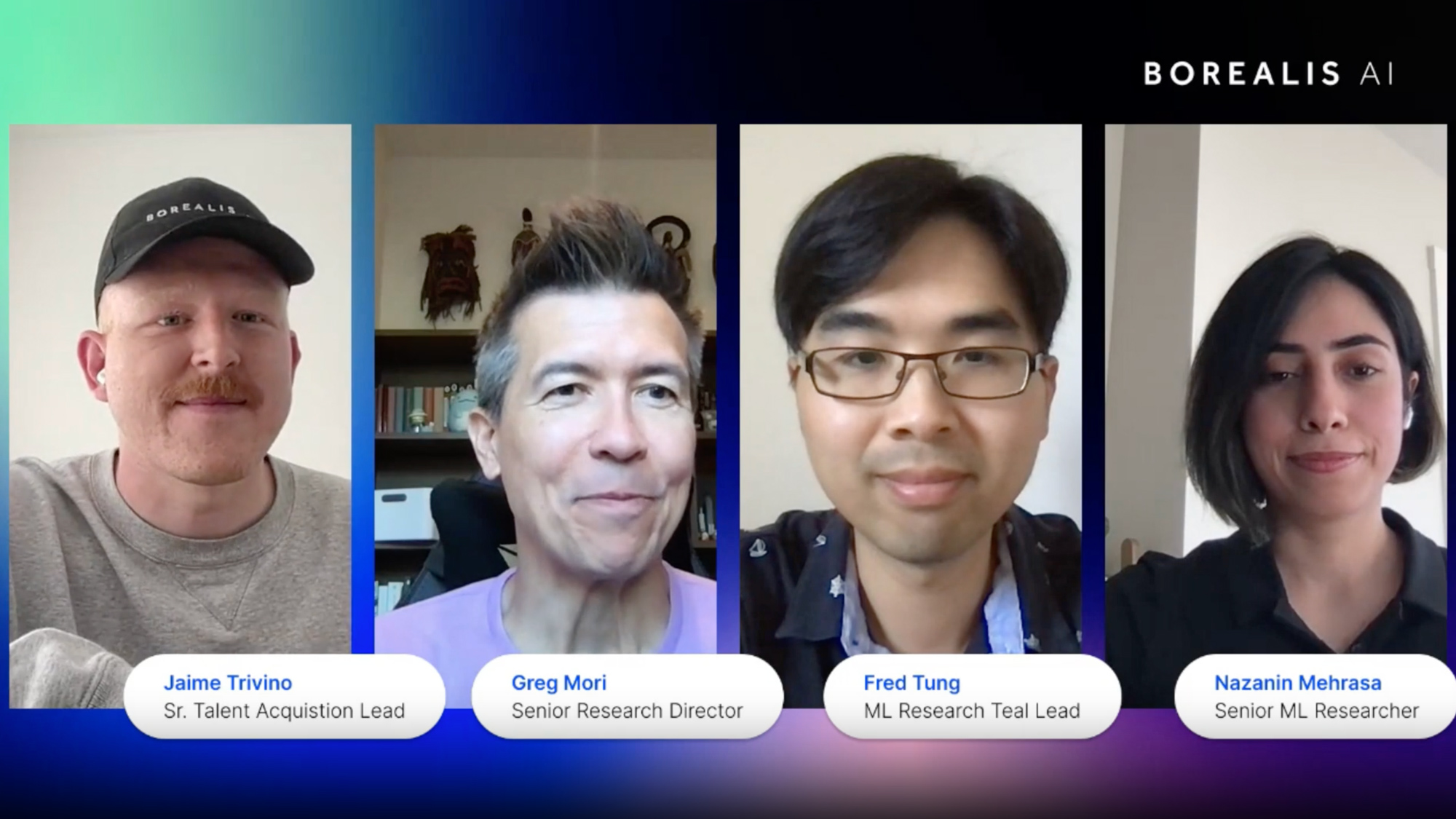
Keeping banking relevant, accessible, and customer-centric requires competitive and innovative AI-based products. So how is Borealis AI turning scientific pursuit into real-world impact? Read on to find out. 👇
Creating impact with science
We’re RBC’s innovation engine. Our team of approximately 40 researchers are leaders in computer vision, machine learning, and natural language processing. Many have PhDs in computer science, physics, computational finance, mathematics and more. We operate as a research institute of the Royal Bank of Canada, conducting research and building innovative machine-learning models for financial services. We collaborate closely with product owners and engineers to translate our research into real-world solutions that improve the lives of RBC’s customers. Our research helps build technologies that respect privacy and upholds principles of Responsible AI. Ensuring AI is being developed and used in an ethical way is a top priority.
At the cutting-edge
We are focused on conducting cutting-edge research that supports capital markets, personal and retail banking, and commercial banking, and as part of RBC, we have access to data, problems, and domain experts to drive our research agenda. Our research aims to advance AI, anchor the development of AI-based products in scientific research and contribute knowledge and resources to the research community.
Our work centers around three main North Star research areas that serve as the main research streams guiding our work: ATOM (Asynchronous Temporal Models), Causmos (Casual Machine Learning from observational data) and Photon (Non-cooperative learning in competing markets).
“Our three North Star directions focus our long-term research and support the products we build across the spectrum of opportunities RBC provides for us.”
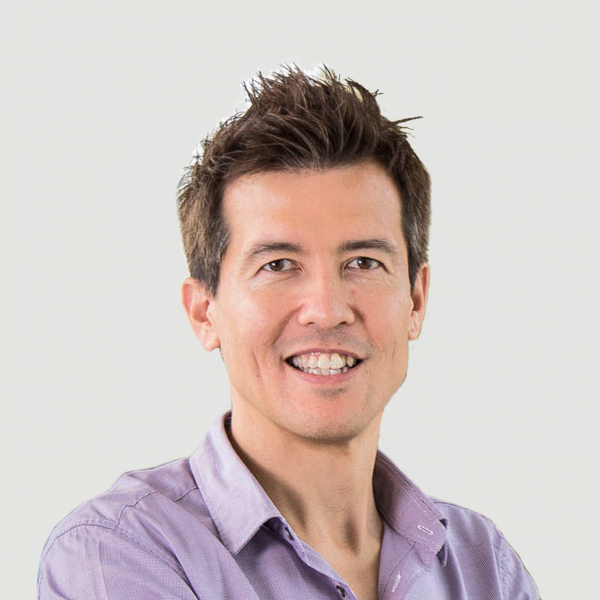
Greg Mori
Senior Director of Research, Borealis AI
ATOM: Asynchronous Temporal Models
ATOM helps RBC uncover reliable insights from piles of disparate data. With ATOM, we build machine learning models capable of making inferences from partially observed, multi-source, asynchronous temporal data. These are the types of data commonly found in banking applications — various kinds of transaction data to client interactions with our banking services. This research supports the work we do with personal banking at RBC. For example, our technology is integrated into RBC’s digital platforms like NOMI Forecast to keep clients connected to their money, offering tailored insights and advice.
Causmos: Casual Machine Learning from observational data
Causmos ensures our products remain robust and relevant to our customers and our industry. This research area focuses on causality research and aims to develop machine learning models that can answer causal questions and generalize out of distribution (OOD). For example, this work helps us gain insights into how our machine-learning models would function if there were changes in macroeconomic conditions, such as GDP growth or fluctuations in the unemployment rate.
Photon: Non-cooperative learning in competing markets
Photon keeps our Capital Markets offering at the cutting edge. Photon supports our work dealing with challenges such as low signal-to-noise ratio, structured prediction, and game theoretic impacts from the decisions we make. You can learn more about how research areas like computer vision are relevant to the work we do in financial services in this blog post.
“Globally, Capital Markets is going through a paradigm shift towards complete automation through AI Systems. So, we believe that a principled understanding of the interactions between statistical models that operate in a common environment will soon be a key success factor for leaders in the field.”
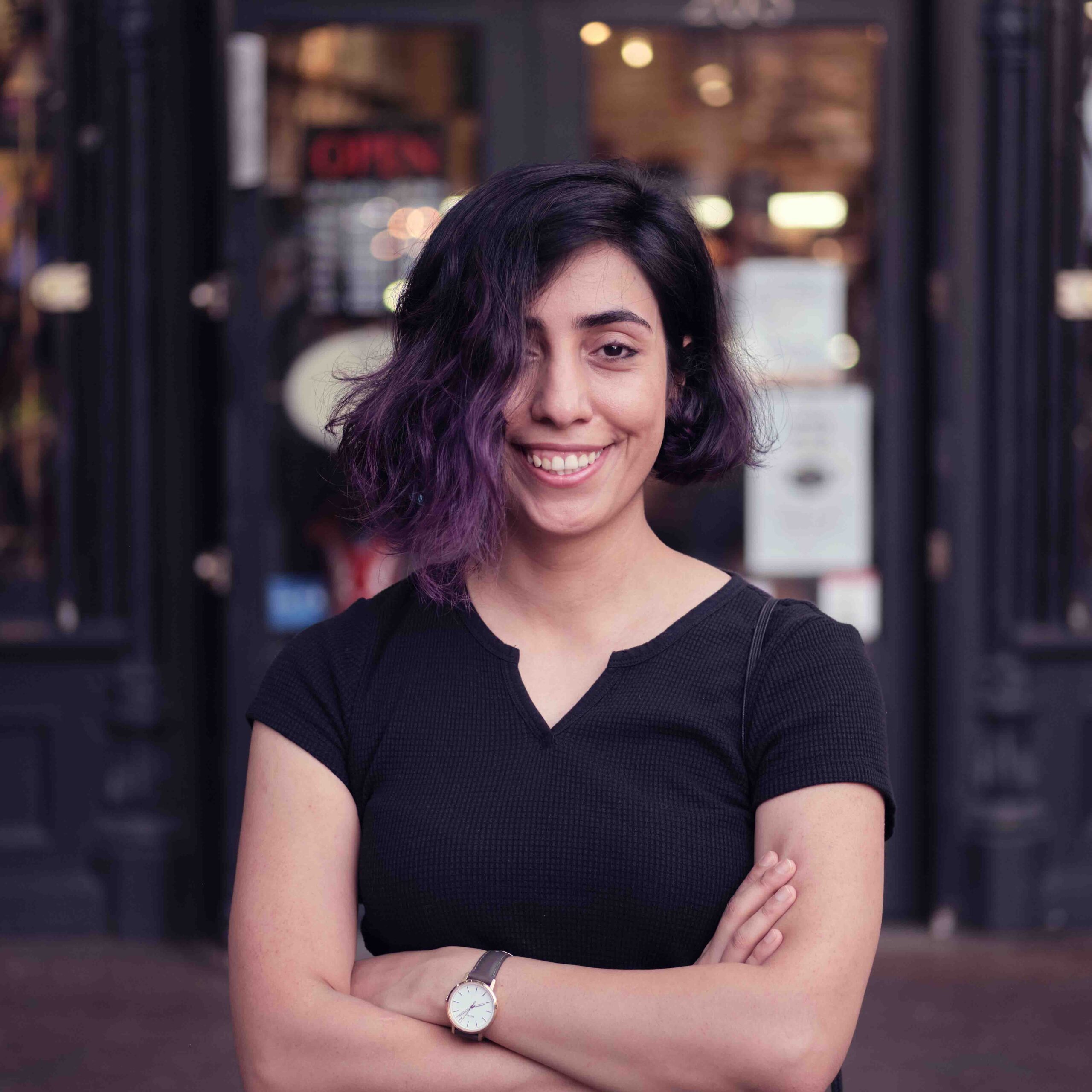
Nazanin Mehrasa
Senior Machine Learning Researcher, Borealis AI
Beyond North Star Research
The best way to drive innovation in the AI community is by sharing our work. As discussed throughout the panel event, our researchers can make a significant scale impact across the bank by bringing to life cutting-edge products for financial services and the academic community. The team has published papers at conferences such as ICML, NeurIPS, TMLR, ECCV and more.
Noteable papers published by our team
RankSim: Ranking Similarity Regularization for Deep Imbalanced Regression
International Conference on Machine Learning (ICML) 2022
Continuous Latent Process Flows
Conference and Workshop on Neural Information Processing Systems (NeurIPS) 2021
Variational Selective Autoencoder: Learning from Partially-Observed Heterogeneous Data
International Conference on Artificial Intelligence and Statistics (AISTATS) 2021
To read more of our work, you can explore our research library at Publications Page.
How to join the Borealis AI Team
Are you aspiring to build a career in AI and ML research? Do you want to join a team that is associated with innovation and high ethical standards? If you are looking for opportunities to make a personal impact, we have several programs and opportunities worth looking into; our Fellowships program, Let’s SOLVE it, Research Internships and current opportunities.
You can also find out more about our North Star Research by checking out our recent LinkedIn panel event.
News
RBC Capital Markets announces the launch of Aiden® Arrival, the second algorithm on the Aiden® platform
News